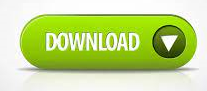
However, the uplift or drop is, in fact, only temporary and is not going to last once you deploy the winning version universally and measure its impact over a significant period. What are some of the errors that creep into your A/B test results? Type I errorsĪlso known as Alpha (α) errors or false positives, in the case of Type I error, your test seems to be succeeding, and your variation seems to cause an impact (better or worse) on the goals defined for the test. Let’s take a closer look at what exactly do we mean by Type I and Type II errors, their consequences, and how you can avoid them. This causes misinterpretation of test result reports, which ultimately misleads your entire optimization program and could cost you conversions and even revenue. Popularly known as Type I and Type II errors, these essentially lead to an incorrect conclusion of tests and/or erroneous declaration of winner and loser. But as test owners, you should not need to bother about this as your tool should take care of this.Įven after following all the essential steps, your test result reports might get skewed by errors that unknowingly creep into the process. Instead, you can only increase the possibility of the test result being true.
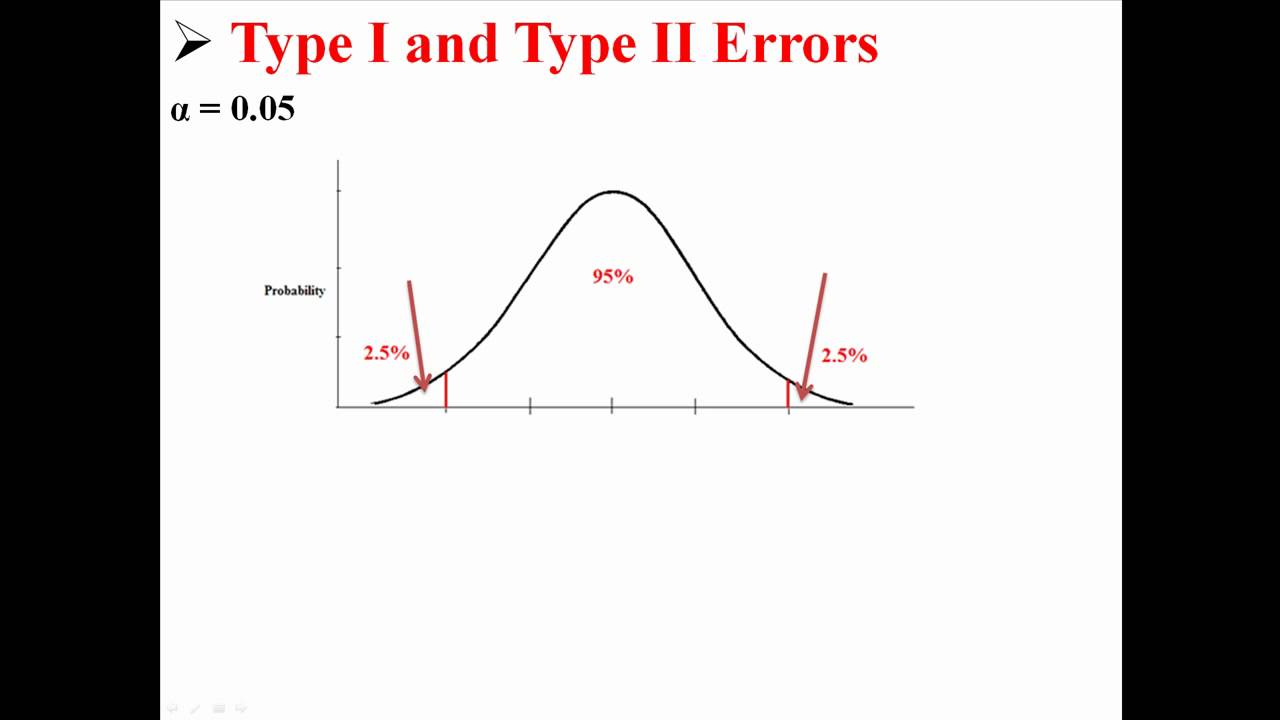

Therefore, you can never be 100% sure of the accuracy of the results you receive or reduce risk to 0%. Statistics is the cornerstone of A/B testing, and calculating probabilities is the basis of statistics. While A/B testing might sound simple,the science and math behind its operation and the computation of the results can get quite tricky. Pretty straightforward, right? Well, not so much. A/B testing involves randomly splitting incoming traffic on your website between multiple variations of a particular webpage to gauge which one positively impacts your critical metrics.
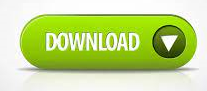